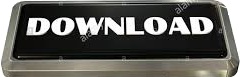
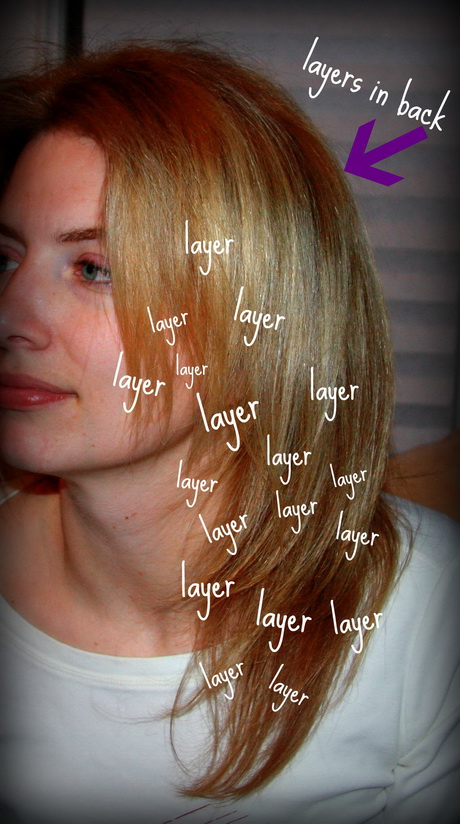
A good illustration of this is a situation where we have a field named “date_of_birth” in the source system which is a date value making up the year, month and date of birth of an individual. For instance, not every field in the source system can be used in the target system the transformation process will look into choosing the precise fields that can be synchronized between the source and the target. During this phase, a number of transformation types are applied.
Easy cut studio vs sure cuts a lot series#
In order to prepare the extracted data for integration into a data warehouse, a series of rules are applied to scrutinize, cleanse, and organize the data to ensure only the ‘fit’ data are loaded. Many of these formats, including relational forms, XML, JSON, flat files, etc., could be used for the data in the source system. After establishing the technical and business requirements, there is a need to understand the right fields/attributes that meet these requirement and also the format in which they are stored. The source of the data we are trying to fetch could be a data storage platform, legacy system, mobile devices, mobile apps, web pages, existing databases etc. Through the use of a set of technical and business criteria, the ETL process makes sure the data is clean and properly organized to meet the needs of business intelligence.Ī typical ETL process employs a step-by-step approach which starts by understanding the structure and semantics of the data contained in the source system. This process is what forms the foundation upon which workflows in data analytics is built.
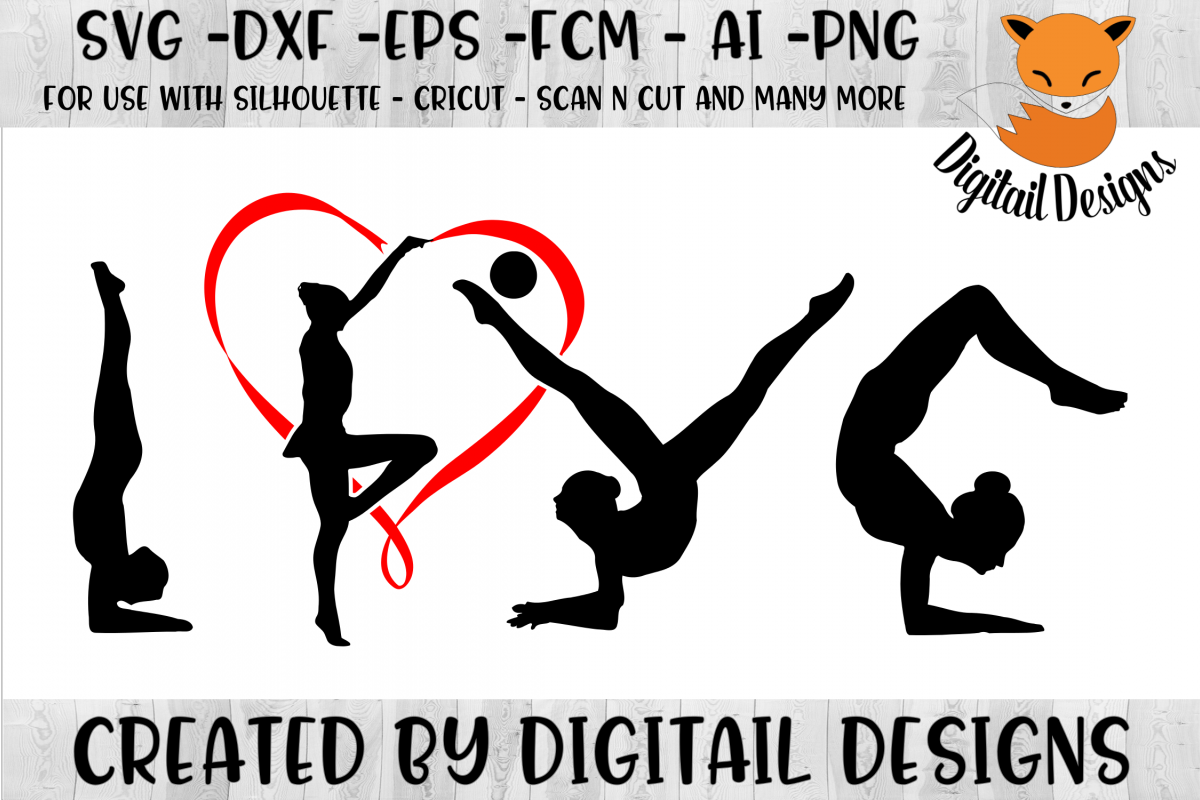
The extract phase of ETL deals with exporting and validating the data, the transform phase involves cleaning and manipulating the data to ensure it fits into the target and the final stage, which is loading involves integrating the extracted and cleaned data into the final destination. ETL in clinical data science/healthcare domainĮxtract, Transform and Load (ETL) is a three-stage process that involves fetching the raw data from one or more sources and moving it to an intermediate, temporary storage known as staging area transforming the extracted data to enforce data validity standards and conformity with the target system and loading the data into the target database, typically a data warehouse or repository.With that said, the rest of the article is organized as follows If you are unfamiliar with the area of clinical data science or you just want a fast refresher on the key ideas, you might want to have a sneak peek of the introductory article. In the first article, I provided a thorough overview of the expansive field of clinical data science, and that sets the foundation upon which this edition is built.
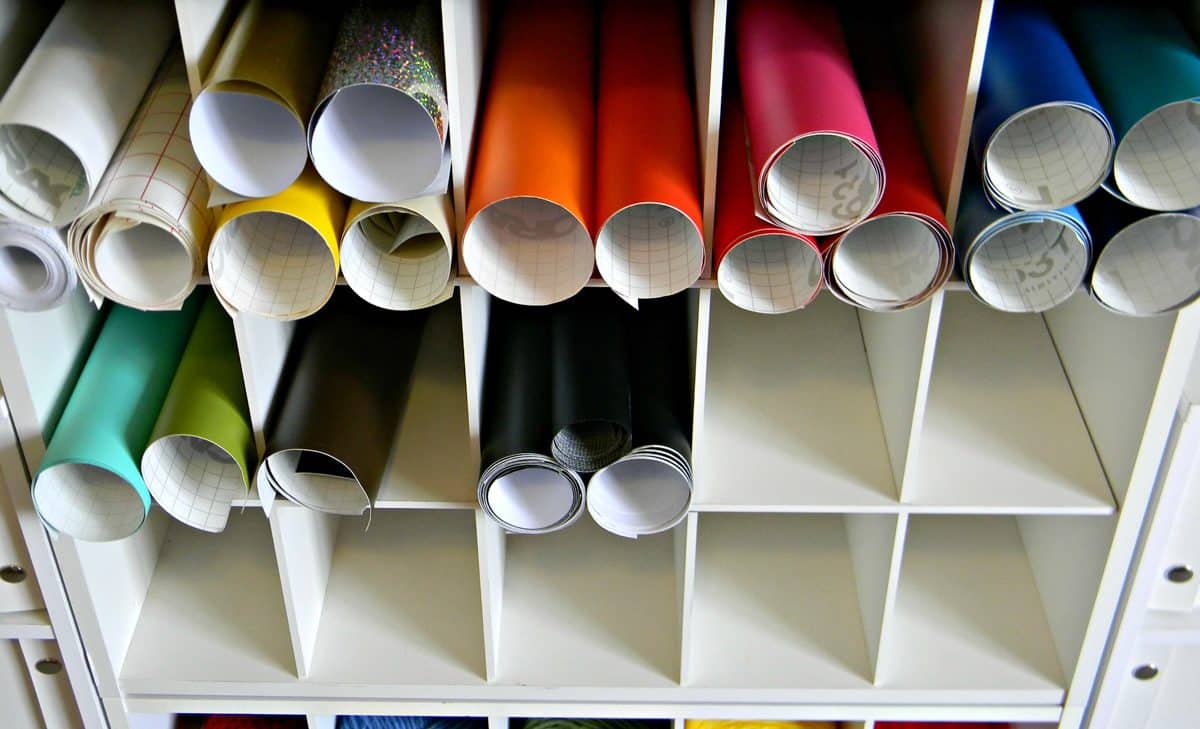
This is the second article in my clinical data science series. An overview of ETL in healthcare, a critical part of the data lifecycle in clinical data science
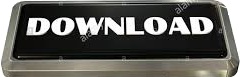